- Everything announced at Apple's WWDC 2025 keynote: Liquid Glass, MacOS Tahoe, and more
- AI活用による保険代理店の変革―人材育成と業務効率化の新戦略とは?
- The FPGA turns 40. Where does it go from here?
- You can dismiss Apple Watch notifications with a flick of your wrist now
- Shortcuts is the best Apple app you're not using - and iOS 26 makes it even more powerful
IoT, edge computing and AI projects pay off for asset-based enterprises
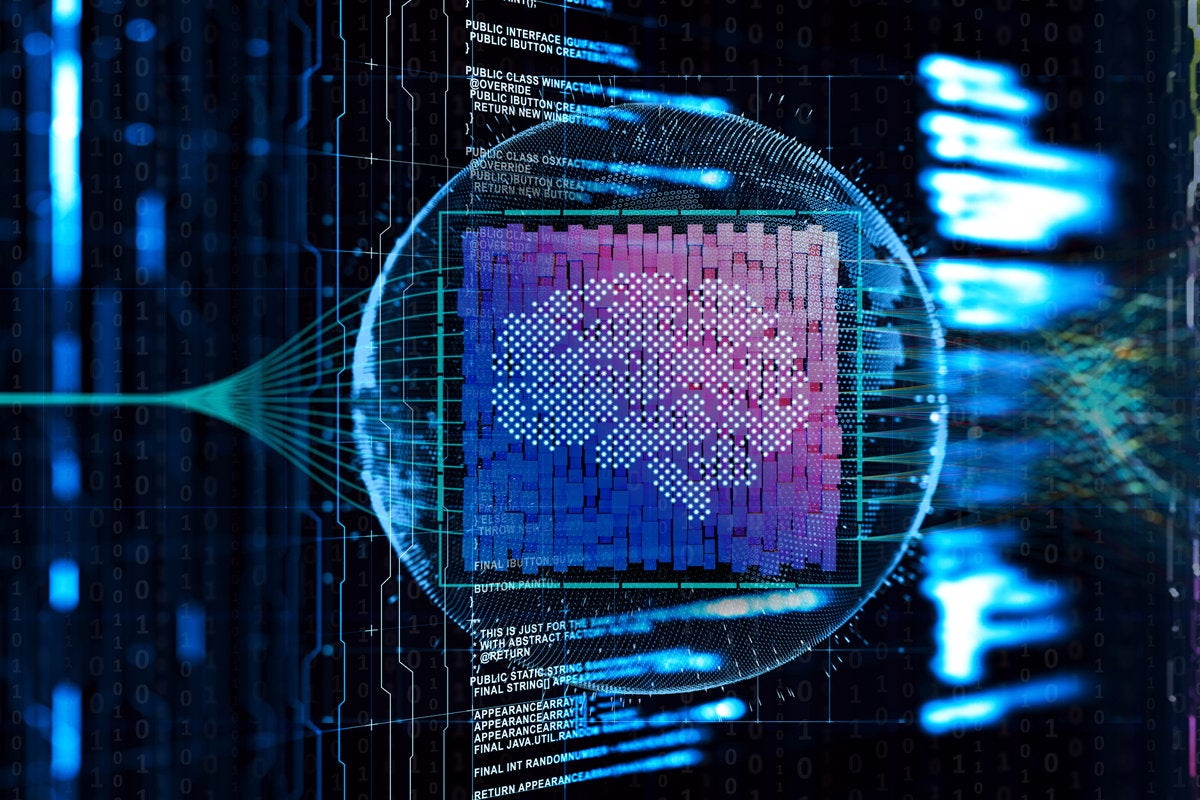
Bill Holmes, facilities manager at the Corona, Calif., plant that produces the iconic Fender Stratocaster and Telecaster guitars, remembers all too well walking the factory floor with a crude handheld vibration analyzer and then plugging the device into a computer to get readings on the condition of his equipment.
While all of the woodworking was done by hand when Leo Fender founded Fender Musical Instruments Corp. 75 years ago, today the guitar necks and bodies are produced with computer-controller woodworking routers, then handed off to the craftsmen who build the final product. Holmes says he is always looking for the latest technological advances to solve problems (he uses robotics to help paint the guitars), and there’s no problem more vexing than equipment breakdowns.
Preventive maintenance, where machines get attention on a predetermined schedule, is insufficient, he says. “Ninety percent of breakdowns are instant failures that shut down processes. That’s hard on business. If you can spot a failure before it happens, you’re not shutting down production and the maintenance team isn’t running around putting out fires.”
With 1,500 pieces of equipment at the 177,000-square-foot facility, Fender is a classic candidate for putting sensors on the machinery and using AI analytics to anticipate failures. That’s exactly what Fender is doing, but with a twist – the company is using Amazon’s cloud-based Monitron service, so all of the data processing take places in Amazon’s cloud.
For smaller companies like Fender, Amazon’s fully managed service is attractive because Amazon provides the wireless sensors, which connect to Amazon’s Wi-Fi gateway over near-field communications (NFC). Amazon’s gateways are preconfigured to send relevant data to the Amazon cloud for analysis. Amazon develops the machine-learning algorithms, processes the data, and sends alerts directly to Holmes.
“They basically brought the price down low enough to where mom-and-pop shops can put this on one of their pieces of equipment and do the monitoring very easily without training. This is huge. Every manufacturer has a critical piece of equipment that will shut down production if it fails,” Holmes says.
So far, Holmes has instrumented nine mission-critical machines, and is planning to deploy the system at a second manufacturing facility in Ensenada, Mexico. Using the cloud provides the additional benefit of enabling Holmes to one day aggregate data from both sites for additional analysis. Plus, he anticipates being able to keep track of both sites from a single dashboard.
How edge computing enables AI
Dave McCarthy, research director for edge strategies at IDC, says that in industries like manufacturing, transportation, logistics, healthcare, retail, oil and gas – basically any industry that has physical assets – machine-generated data is “the wind in the sails of edge computing.” He adds, “Finding meaningful insight in the data coming off those machines and automating the responses to that data is the AI story.”
The general rule of thumb is that performing AI processing at the edge is best suited for real-time, latency-sensitive applications that wouldn’t operate efficiently if those large data sets had to be shipped to the cloud, says Tilly Gilbert, senior consultant at STL Partners. In addition to the latency issue, edge computing reduces backhaul costs and helps companies comply with privacy regulations and security policies that might be violated if sensitive data were sent offsite.
AI-driven data processing at the edge is moving beyond niche cases and is becoming more mainstream, driven by the twin business needs for increased uptime and improved performance, McCarthy says
A number of factors are coming together to make edge/AI easier to deploy, including the proliferation of physical assets that are preconfigured with IoT sensors, and the increasing number of vendors that are offering edge technology. These include systems integrators, third-party startups, the hyperscale cloud providers as well as traditional infrastructure players that position the edge as an extension of the data center.
For enterprises, that lets them workloads run in the most appropriate location, whether that’s on-prem, in the cloud or at the edge. Or a combination – as the Fender example demonstrates, there are a variety of ways to mix and match technologies and approaches to get the best of both the edge and cloud worlds.
Just as most enterprises these days are operating in a hybrid cloud or multi-cloud environment, AI-based edge applications don’t run in isolation, McCarthy points out. Even if AI processing is occurring at the edge, the machine-learning algorithms were probably developed and the models were trained in the cloud. And that real-time data can be rolled up and aggregated into the cloud to enable analysis of historical data sets that can guide longer-term planning.
AI at the retail edge
The most exciting aspect of the edge/AI combo is that it enables new applications, Gilbert says.
Because many enterprises don’t have the skills to develop AI analytics capabilities in-house, or may not even be aware of some of the possible use cases, start-up third parties are taking a lead role in developing and deploying ready-made systems. For example, major retailers like Walmart and Kroger are both rolling out AI-based edge systems at the self-checkout lanes of their stores in order to reduce loss due to customers either inadvertently or intentionally not paying for everything in their shopping cart.
Alex Siskos, vice-president of strategic growth at Irish startup Everseen, which is providing the technology to both Walmart and Kroger, says his company has been able to address a previously intractable problem for retailers: shrinkage or loss. He says retailers knew they were losing money at the self-checkout, but had no way to tell if it was from honest mistakes by customers, by ‘sweethearting,’ where employees give away merchandise to friends, or by clever thieves who, for example, might place a stick of gum under a larger, more expensive item so the scanner charges the customer only for the gum.
Everseen strategically places GPU-powered, computer-vision cameras at the self-checkouts and has developed software that integrates with the retailer’s scanning systems, so if the scanner says ‘stick of gum’ but the camera sees ‘box of diapers,’ a variety of actions can be triggered in real time. The customer might get a pop-up alert on the check-out display screen that says something like, ‘the machine may have mis-scanned that last item.’ The idea is to give customers the benefit of the doubt and allow them to self-correct before intervention by an employee is required. As a last resort, the system has the ability to replay video of the act in question right on the self-checkout display screen.
“We are able to turn unstructured data into insight, action and ultimately profit,” Siskos says. He estimates that retailers are saving between $2,500 and $4,500 per store per week from theft reduction and improved inventory accuracy.
The Everseen system processes data at the edge because, as Siskos says, “that’s where the action is, that’s where the moment of truth is.” The fully integrated offering consists of Dell PowerEdge servers running the Everseen software, which is written on top of a development platform created by GPU-provider Nvidia. But there are cloud components as well; the models are trained in the cloud, and the management and monitoring occur in the cloud.
In addition, Everseen currently monitors more than 100,000 checkout lines in the U.S. and Europe and culls 4-5 second clips of those ‘moments of truth’ where items were scanned incorrectly. That select data is sent to the cloud for reporting purposes, as well as to help train the algorithms. “AI is a hungry animal,” Siskos says. “The more you feed it, the better it gets.”
AI gains traction in healthcare
Healthcare is another area in which edge computing is powering AI.
Dr. Andrew Gostine is an anesthesiologist and entrepreneur who created a company that applies AI to optimize hospital resources in order to boost efficiency and save money.
Hospitals save lives, but they are also a business. Just as restaurants need to turn tables and seat as many parties as possible during the course of a day, hospitals need do the same with surgical suites. Gostine’s company, Artisight, uses multiple wireless cameras mounted in surgical rooms to act as “air traffic control.” For example, the moment the patient is wheeled into surgery, the anesthesiologist and surgeon are automatically notified. There’s also a large display screen in the hallway outside the operating room, similar to what you’d see in an airport telling flyers the status of their flight and what gate to go to, that helps to make sure hospital staffers are in the right place and the right time.
Sounds pretty simple, but Gostine says his system is delivering 16% productivity gains at the hospitals in the Chicago area where it is being deployed. The Artisight system is built on Nvidia’s Clara Guardian edge/AI platform for hospitals and is delivered in a pre-packaged bundle that runs on Dell servers and storage. Processing is done on site because the volume of data – Northwestern Memorial Hospital produces 1.2 petabytes of video per day – would be far too expensive to send to the cloud and would also create latency issues, says Gostine.
The Artisight system scrubs people’s identities to preserve their privacy. It also records the key parts of the operation so that surgeons can go back and study their performance and share the videos with their peers to get feedback.
Gostine says the technology can be used in an ever-expanding number of edge use cases. For example, cameras can monitor a patient room to detect if the patient gets out of bed and falls. The system can also monitor patient rooms as part of a capacity management program – in other words, notifying housekeeping immediately when a room is vacated, keeping an inventory of available rooms, making sure the linens have been changed and that the right medical equipment is in the room.
Everyone who follows AI is aware of IBM’s bold prediction that Watson would one day cure cancer, only to have that project fail to deliver results. Gostine argues that over-promising “miracle cures” has set AI back. More important, he says, is using AI for applications that might be more mundane, but more practical, and can improve efficiency and cut costs, which ultimately frees up hospital resources that can used to expand patient care.
Copyright © 2021 IDG Communications, Inc.